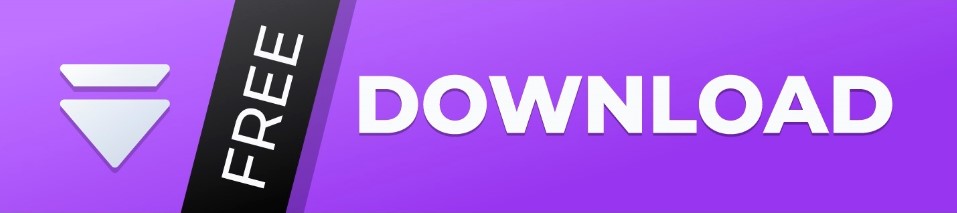
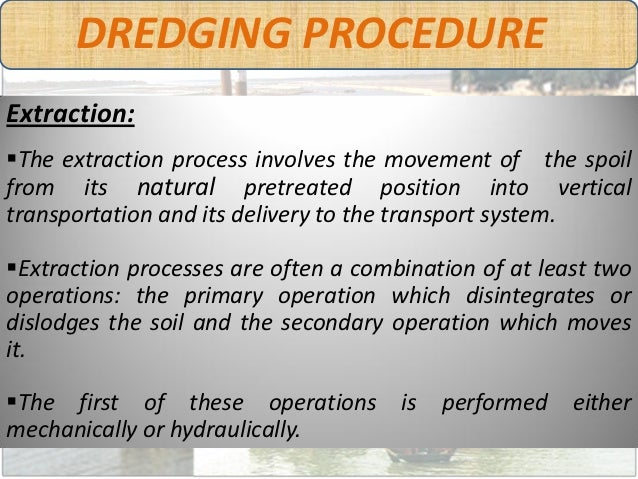
This level of risk is called the significance. Conventional tests of statistical significance are based on the probability that a particular result would arise if chance alone were at work, and necessarily accept some risk of mistaken conclusions of a certain type (mistaken rejections of the null hypothesis). The process of data dredging involves automatically testing huge numbers of hypotheses about a single data set by exhaustively searching - perhaps for combinations of variables that might show a correlation, and perhaps for groups of cases or observations that show differences in their mean or in their breakdown by some other variable.
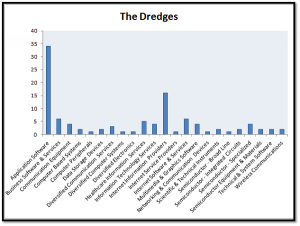
Examples in meteorology and epidemiology.Hypothesis suggested by non representative data.Data dredging (also data fishing, data snooping, and p -hacking) is the use of data mining to uncover patterns in data that can be presented as statistically significant, without first devising a specific hypothesis as to the underlying causality.
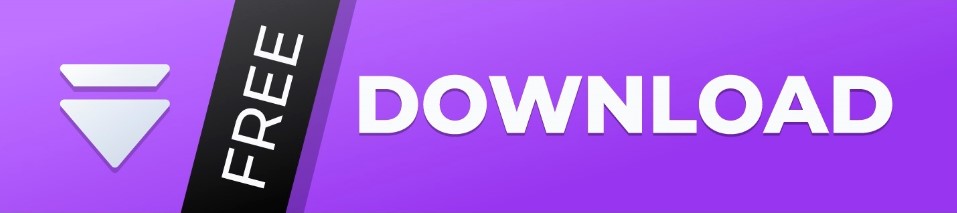